Advanced Guide to OTT Content Personalization: Building Smart Recommendation Systems
- Didem Sübar
- Mar 27
- 3 min read
Updated: 7 days ago
Personalization matters more than ever, with COVID-19 and the surge in digital behaviors raising the bar. Three-quarters of consumers switched to a new store, product, or buying method during the pandemic.
As we navigate through 2025, the abundance of content has made sophisticated personalization systems the cornerstone of successful OTT platforms. According to research by Epsilon, 80% of consumers are more likely to make a purchase when brands offer personalized experiences.
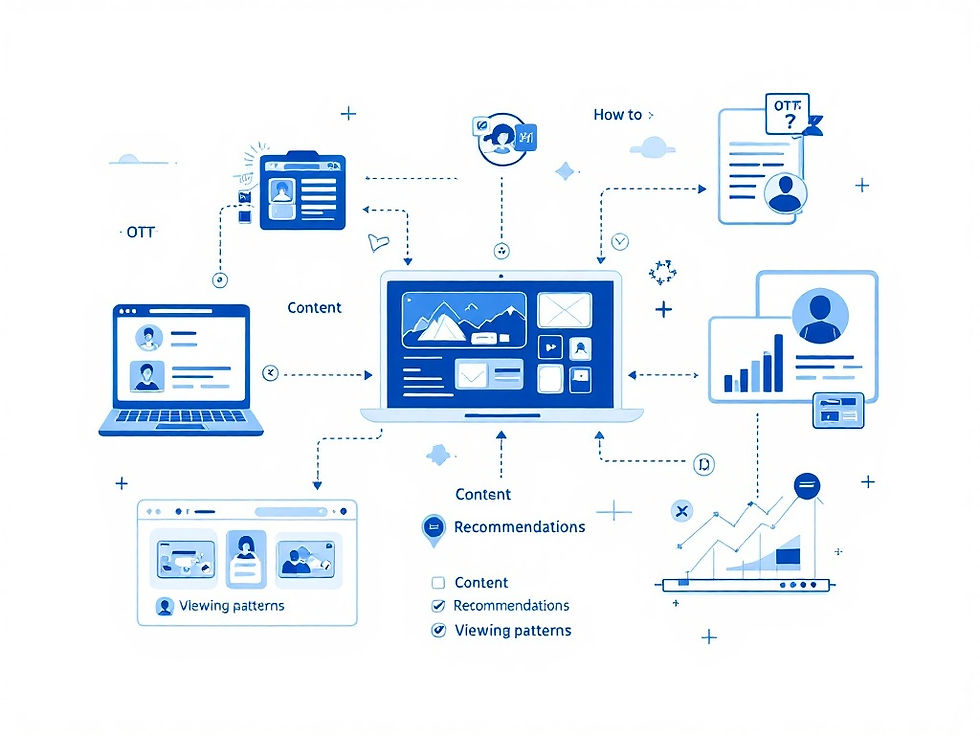
The foundation of effective OTT personalization rests on three primary pillars: data architecture, behavioral analysis, and content intelligence. Modern streaming platforms process an average of 500 data points per user session, transforming raw viewing patterns into actionable insights. This intricate dance of data and algorithms creates a viewing experience as unique as each subscriber's preferences.
At the heart of personalization lies a robust data collection framework, functioning as a digital nervous system that captures and processes everything from viewing history to content interaction metrics. This framework extends beyond simple data collection, incorporating sophisticated device usage behavior analysis and time-based engagement data to create a comprehensive understanding of viewer preferences.
Modern OTT platforms employ sophisticated behavioral analysis tools that transcend simple viewing history. These systems delve deep into content consumption patterns, analyzing when and how viewers engage with content, their genre-switching behavior, and their content discovery paths. This detailed analysis enables platforms to create highly accurate viewer profiles that evolve with changing preferences.
The recommendation engines powering today's personalization systems utilize advanced machine learning algorithms that continuously evolve. These systems have achieved remarkable accuracy rates, with leading platforms reporting up to 89% accuracy in predicting viewer preferences. The technology adapts to seasonal viewing changes, accounts for cultural and regional preferences, and learns from collective viewing patterns to create an ever-improving recommendation system.
Contemporary collaborative filtering has moved far beyond basic "users who watched X also watched Y" models. Current systems incorporate multi-dimensional similarity metrics, considering temporal viewing patterns, social influences, and cultural context. This sophisticated approach enables platforms to provide recommendations that feel both personal and contextually relevant.
Implementing effective personalization requires a carefully planned architecture that includes scalable data processing infrastructure, real-time recommendation engines, and comprehensive content metadata management systems. The data pipeline forms the backbone of this architecture, processing viewer interaction tracking, content consumption metrics, and user preference signals in real-time to generate meaningful insights.
Success in OTT personalization can be measured through various performance indicators. Leading platforms typically aim for a 30% improvement in engagement rates, 40% reduction in content discovery time, and recommendation acceptance rates exceeding 65%. These metrics directly correlate with increased customer lifetime value and reduced churn rates.
Looking toward the future, the personalization landscape continues to evolve with emerging technologies such as AI-driven content tagging and emotional response analysis. Cross-platform preference synchronization and contextual recommendation systems are becoming increasingly sophisticated, while predictive content licensing helps platforms optimize their content offerings based on anticipated viewer preferences.
The implementation roadmap for successful personalization begins with establishing a solid foundation of data infrastructure and basic recommendation systems. This foundation enables platforms to progress to more advanced features like machine learning algorithm integration and real-time personalization. The final optimization phase focuses on advanced analytics integration and predictive modeling for continuous improvement.
Maximizing personalization effectiveness requires a commitment to robust data collection, gradual feature rollout, and regular algorithm updates. Successful platforms maintain transparency with users while continuously refining their personalization systems based on performance metrics and user feedback.
As we progress through 2025, OTT content personalization represents the future of streaming engagement. The platforms that master personalization will lead the industry in viewer satisfaction and retention. The key lies in building systems that not only recommend content but truly understand and anticipate viewer preferences, creating a viewing experience that feels uniquely tailored to each individual user.
For streaming providers looking to transform their OTT platform with advanced personalization, VUCOS offers cutting-edge solutions tailored to specific platform needs. Our team of experts specializes in implementing smart recommendation systems that drive engagement and growth, helping platforms stay competitive in an increasingly personalized streaming landscape.
Comentarios